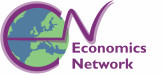
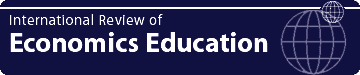
A Comparison of Academic Performance in A-Level Economics between Two Years
Ray Bachan and Barry Reilly(note 1)
International Review of Economics Education, volume 2, issue 1 (2003), pp. 8-24
DOI: 10.1016/S1477-3880(15)30154-7 (Note that this link takes you to the Elsevier version of this paper)
Up: Home > Lecturer Resources > IREE > Volume 2 Issue 1
Abstract
Performance equations are estimated using ALIS data for two cohorts of students in England and Wales taking Economics A-level examinations in 1998 and 2000. The approach adopted uses an ordered probit model and the empirical results confirm some established research findings in the educational literature. In particular, we find that prior attainment at GCSE level and performance in GCSE Mathematics exert a strong influence on A-level achievement in Economics. However, we also find a significant gender differential in performance in both years. A counterfactual exercise was implemented and this established that standards within the subject appear to have remained relatively constant across the two years in question.
JEL Classification: H75, A21
Introduction
The number of students studying Economics at A-level(note 2) in England and Wales declined by over 50% between the years 1992 and 2000 (DfEE, various years). Several explanations have been adduced to account for this declining trend in enrolments. The cited reasons include the increased number of competitor subjects at A-level (e.g. Business Studies), the abstract, mathematical and numerical nature of the subject, the core curriculum offered at GCSE (which excludes Economics), and the perception that a relatively severe grading policy is adopted by examiners within the subject. Ashworth and Evans (1999), Hirschfeld et al. (1995), Williams et al. (1992) and Horvath et al. (1992) provide evidence on some of these issues. The perceived relative difficulty of a subject deters many potential candidates, and Fitz-Gibbon and Vincent (1994) classified Economics as a relatively ‘difficult’ subject using a variety of methodologies, including subject-pair analysis and reference tests. Ashworth and Evans (2000) confirm the challenging nature of Economics at A-level and, more recently, Reilly and Bachan (2004) outlined an econometric methodology to quantify the degree of ‘difficulty’ of A-level Economics relative to the close competitor subject of Business Studies. Goldstein and Cresswell (1996) have criticised quantitative approaches to the issue of subject difficulty on conceptual and technical grounds. They contend that quantitative methods used to determine relative subject difficulty make assumptions that are difficult to substantiate, and argue that qualitative judgements are more appropriate and better grounded in theory. In particular, it is suggested that only the judgement of ‘experts’ (i.e. examiners themselves) leads to more meaningful conclusions (see Cresswell, 1996). Goldstein’s and Cresswell’s views have been challenged in the educational literature. For instance, Fitz-Gibbon and Vincent (1997) argue for a need to investigate subject difficulty using a sound baseline measure (GCSE scores). Their central point is that the question still remains as to why students of similar ‘ability’ are awarded different grades in different A-level subjects. We concede that the purpose of public examination may not be one-dimensional, but the final examination grade is important to certificate users as it reflects what is taken to be a ‘common currency’.
In this short paper, we examine the key factors that determine student performance in Economics at A-level, and undertake a comparative analysis of performance in A-level Economics between two years. Our primary concern is to establish whether A-level Economics was broadly comparable in terms of ‘difficulty’ between 1998 and 2000. These years are chosen because they represent a period in which the syllabi offered by the major examination boards remained broadly constant in terms of their content and assessment patterns. We explore the counterfactual of what the A-level grade distribution for a sample of Economics candidates who took the examination in 2000 might have been had they taken the examination in 1998. The converse exercise is also implemented. This then allows us to compute an average grade adjustment that standardises for difficulty across the two years using the familiar UCAS points tariff.(note 3)
The structure of this paper is now outlined. The next section describes the dataset used in our analysis and it is followed by a section containing a description of the econometric methodology employed. The penultimate section reports the empirical results and a final section provides a summary of conclusions.
Data
The data used are obtained from the A-Level Information System (ALIS) project administered at the Curriculum, Evaluation and Management Centre (CEM centre) at Durham University. The specific data employed in this study are based on academic performance by a sample of Economics candidates in the 1998 and 2000 examinations.
The dataset for the 1998 examinations comprised 70,121 A-level and Advanced GNVQ(note 4) students. From these data, 43,910 students studying two or more A-levels (excluding General Studies and Advanced GNVQ) were obtained. Once allowance was made for missing values, clean information on 2,086 Economics students was obtained. This comprised 1,204 male candidates and 882 female candidates. The dataset for 2000 comprised 82,063 students from which 2,019 Economics candidates were available composed of 1,230 male and 789 female students.(note 5)
The set of independent variables includes measures of prior attainment (average GCSE scores), gender, ethnicity, school type, parental characteristics, examination board and other A-levels studied. It is important to note that, for reasons of confidentiality, the data are limited in some respects. It is not possible to identify either schools or colleges by their names or postcodes. It is therefore not possible to assign certain factors (e.g. location, funding, staff/pupil ratios, numbers on roll, teacher or class characteristics and processes, and the number of teaching sets per college) to the individual-level data used here. In addition, it did not prove possible to identify prior attainment in GCSE Economics (if taken) for the sample of students used here. We were also unable to differentiate between modular and linear courses from the available syllabus information. Moreover, due to data coding, it was not possible to distinguish between the NEAB and the AEB examination boards. For the purposes of this exercise, they are amalgamated with AQA following their merger in 2000. The examination boards are grouped into AQA, Edexcel and OCR to make the 1998 sample comparable to that of 2000. The summary statistics for the 1998 sample and the 2000 sample are reported in Table A1 of the appendix.
As a preliminary exercise, it is useful to examine some of the key characteristics of the two samples. In general, there is no substantial difference in A-level performance in terms of specific grade categories between the two years and the sample averages are broadly in line with national figures for Economics in these years (DfEE, various years). The overall chi-squared value suggests some variation in performance between 1998 and 2000, but the result is largely driven by changes at the bottom end of the distribution, where the proportion failing Economics fell by 3.6 percentage points between 1998 and 2000.
The major differences between the sample cohorts in terms of the other variables are in average GCSE scores, GCSE mathematics grades, examination boards and the type of school attended. The sample of Economics candidates for 2000 appears better qualified than their earlier counterparts using the average GCSE performance measure. The average differential in GCSE scores is statistically significant and suggests an average advantage for the 2000 sample that is close to 0.15 of a GCSE grade point. This may be taken as indicative of a quality differential between the two cohorts of students. The proportion of students awarded a grade A/A* in GCSE mathematics is higher in 2000 than in the earlier year.
A significant shift in the proportion of students entered through different examination boards is also noted. The proportion of students entered through Edexcel declined by almost 12 percentage points in 2000 as compared to 1998, while the proportion of students entered for the OCR examination showed a 9-percentage point increase over the same period. By 2000, AQA appears to be the dominant board in our sample, catering for just over two-fifths of all Economics candidates.
There are significant differences in the proportion of candidates entered for each examination by school type. In particular, there has been a significant decline in the proportion of students entered through further education and sixth-form colleges. In addition, there are significantly more students entered for A-level examinations in Economics from LEA and grant-maintained schools in 2000. There is no significant difference between the ethnic mix of candidates between 1998 and 2000.
Methodology
The empirical methodology used to model grade performance follows Reilly and Bachan (2004). An ordered probit is employed with responses coded 0 (for N/U) to 5 (for A).(note 6) Let yi* denote an unobservable variable that captures the performance level of the ith individual, and let yi denote its observable counterpart coded 0, 1, 2, 3, 4, 5 on the basis of A-level performance. The performance level can be expressed as a function of a vector of explanatory variables (Xi) using the following linear relationship:
It is assumed that yi* is related to the observable ordinal variable yi as follows:
yi= 0 if –∞ < yi* < θ0
yi= 1 if θ0 ≤ yi* < θ1
yi= 2 if θ1 ≤ yi* < θ2
yi = 3 if θ2 ≤ yi* < θ3
yi= 4 if θ3 ≤ yi* < θ4
yi = 5 if θ4 ≤ yi* < +∞
It is clear from the above that the first and the jth intervals are open-ended, so for j = 0, Φ(θj – 1) = Φ(–∞) = 0 and for j = 5, Φ(θj) = Φ(+∞) = 1. If the X vector contains a constant term, then the remaining set of threshold parameters [θ0, θ1, θ2, θ3, θ4] are not identified. The exclusion of a fixed threshold term facilitates an arbitrary location for the scale of yi*. The normalisation adopted in this paper is θ0 = 0. As we can only identify the parameters of the ordered probit up to some factor of proportionality, we also impose the normalisation that σ2 = 1. This restriction simply converts the ui variable to a standard normal variate and the probabilities remain unaffected by the normalisation. In general terms, the ordered probit model can be expressed as:
where Φ denotes the cumulative distribution function of the standard normal.
The general expression for the log-likelihood function of this particular model is then given by:

where wij = 1 if the ith candidate is in the jth category and zero otherwise, and loge is the natural logarithm. Conventional algorithms can be employed to provide maximum likelihood estimates for the β parameter vector and the four threshold parameters [θ1, θ2, θ3, θ4].
The variance–covariance matrix of the ordered probit model is corrected for heteroscedasticity of an unknown form and for the clustering of candidates by educational institution. Ignoring the clustered nature of the data in this case may lead to an understatement of the estimated standard errors. The educational institution represents the only other level available to us in these data and a more detailed multilevel analysis is thus not feasible.(note 7)
In order to explore changes over time in the degree of difficulty of A-level Economics, we undertake a simulation exercise predicting the grade distribution of the sample of candidates for 2000 using the estimated coefficients from the 1998 equation. A reverse simulation exercise, using the sample of 1998 candidates with the 2000 coefficients, is also implemented.(note 8) These two counterfactual exercises allow us to determine if there are sizeable changes between actual outcomes in a given year and what would be predicted if the grade distribution and structure from another year were imposed. For convenience the UCAS points tariff is the metric used to summarise the average grades.
Empirical results
The estimated coefficients(note 9) for each of the performance equations are reported in Table 1.(note 10) The estimated coefficients can also be translated into probability effects using appropriate formulae (see Greene, 2000, pp. 876–7) and this conversion is adopted in certain circumstances.(note 11)
In contrast to Ashworth and Evans (1999) we find a ceteris paribus gender differential in performance in A-level Economics. In both years, females are found to be less likely than males to achieve the highest grade and more likely to fail. This effect was found to be greater in magnitude in 2000.(note 12) Selected interaction terms allowing for variation in gender effects across school type (i.e. grant-maintained institutions) and one A-level subject (i.e. an arts subject) were introduced into the 1998 performance equation. Females in grant-maintained institutions, on average and ceteris paribus, were found to do less well than their male counterparts, but male students taking an A-level in an arts subject were found to do less well than females taking comparable subjects.
A significant effect is also noted for the GCSE variable in both years. A unit increase in the GCSE score raises the standardised ordered probit index by over 1 standard deviation in both years. There is also a significant gender differential in the effect of GCSE scores on performance in 2000. In particular, a unit increase in the overall GCSE score raises, on average and ceteris paribus, the female A-level performance by 1.33 standard deviations, but raises male performance by only 1.33 – 0.18 = 1.15 standard deviations. This particular result suggests that although the performance of males in 2000 is better than females, ceteris paribus, the value-added effect is higher for females. These results also confirm established research on the importance of prior achievement in student attainment at A-level (see, for example, Fitz-Gibbon and Vincent, 1994; O’Donoghue et al., 1997; Yang and Woodhouse, 2001; and Fielding et al., 2003).
It is generally agreed that knowledge of basic mathematics enhances the study of Economics. This is evident in the results for both years. For instance, a student with an A/A* in GCSE mathematics is more likely to achieve a grade A, on average and ceteris paribus, relative to the omitted category. The point estimate implies that the more qualified students, in terms of GCSE mathematics, are about 7 percentage points more likely to achieve a grade A, and about 2 percentage points less likely to fail in both years.
No significant effects were detected for the school controls other than for grant-maintained institutions in 1998. The lack of significance in the estimated coefficients on the sixth-form college, further education college and private school controls, runs counter to the results found in studies using national datasets employing the multilevel methodology. School controls used in these studies were found to exert some significant influence on student performance (see, for example, O’Donoghue et al., 1997; Yang and Woodhouse, 2001). However, these studies are conducted at an aggregate level and do not differentiate between courses of study. The recent study by Fielding et al. (2003), which focuses on A-level performance in chemistry and geography, also finds some evidence of institution type exerting a significant influence on performance. It is suggested that different institutions attract different cohorts with different average GCSE scores: for instance, students attending further education colleges on average have a lower average GCSE score compared to students who attend an independent or grant-maintained school (see Yang and Woodhouse, 2001). These compositional effects do not seem to play a significant role in the present study, as no relevant interaction terms are supported by the data. Indeed, the average GCSE scores of students in different institutions, in both years, are broadly similar and close to the average GCSE scores reported in Table A1. This could also suggest some commonality with regard to the admissions policy adopted by institutions, where a minimum average GCSE score is operative for the study of A-level Economics.
In terms of the set of examination board variables in 1998, students following syllabi for boards captured by the included variables perform statistically better than students following the AQA (AEB and NEAB) base. For instance, students entered for the examination administered by Edexcel were, on average and ceteris paribus, 5 percentage points more likely to achieve a grade A and 2 percentage points less likely to fail compared to the AQA base. A comparable effect was not detected for 2000. Students following OCR performed better than their counterparts following AQA in both years.
Our analysis suggests that the combination of Economics study with an A-level in either a social science or a humanities subject enhances performance. The converse is the case for students who combine Economics with an arts subject or a modern language. It is also interesting to note that there were no significant effects on performance recorded for students combining A-level Economics with A-level mathematics. This may be attributable to the development of a less formal syllabus in Economics. However, the effect for A-level mathematics may be attenuated by the inclusion in the specification of measures that capture student performance on GCSE mathematics.
In terms of parental characteristics, there are no significant effects for 1998 except for those fathers holding further educational levels. The effect associated with this variable lowers the standardised ordered probit index by almost one-sixth of a standard deviation as compared to the base category. There are more significant effects in 2000 and these are largely confined to a father’s economic status and a mother’s educational level. Finally, there were relatively few well-determined effects associated with the ethnic background variables in both years. An exception is a significant effect detected for the Chinese control in 1998. The point estimate suggests that Chinese students taking Economics in 1998 were 10 percentage points less likely to achieve a grade A, on average and ceteris paribus, compared to their white counterparts. However, this effect was not present in the data for 2000.
Table 1 Maximum likelihood ordered probit estimates for A-level performance between 1998 and 2000
Variable | Economics 1998 | Economics 2000 |
Constant | –6.123 (0.304)*** | –7.194 (0.439)*** |
Male | 0.252 (0.063)*** | 1.425 (0.449)*** |
Ethnic background | ||
White | ƒ | ƒ |
Black | –0.020 (0.145) | –0.021 (0.178) |
Asian | –0.053 (0.105) | 0.129 (0.117) |
Chinese | –0.492 (0.183)*** | –0.212 (0.236) |
Other | 0.100 (0.148) | 0.109 (0.141) |
Mother tongue – English | –0.087 (0.120) | 0.148 (0.104) |
GCSE background | ||
Average GCSE score | 1.204 (0.051)*** | 1.328 (0.070)*** |
GCSE score × male | † | –0.177 (0.072)** |
GCSE Maths – A/A* | 0.361 (0.098)*** | 0.266 (0.108)** |
GCSE Maths – B | 0.122 (0.072)* | –0.077 (0.075) |
GCSE Maths – C | ƒ | ƒ |
GCSE Maths – D | –0.201 (0.275) | 0.223 (0.112) |
School type | ||
LEA | ƒ | ƒ |
Grant-maintained | –0.296 (0.159)* | –0.160 (0.113) |
Private | –0.067 (0.104) | –0.096 (0.114) |
Sixth-form college | –0.040 (0.124) | –0.169 (0.121) |
Further education college | –0.041 (0.116) | –0.045 (0.138) |
Grant-maintained × male | 0.438 (0.182)** | † |
Parental characteristics | ||
Mother’s status: | ||
Deceased | –0.171(0.257) | 0.039 (0.219) |
Retired | 0.042(0.192) | –0.106 (0.309) |
Unemployed | –0.088(0.198) | 0.087 (0.180) |
Employed full-time | ƒ | ƒ |
Employed part-time | 0.037 (0.057) | 0.104 (0.062)* |
Self-employed | –0.022 (0.098) | –0.021 (0.105) |
Student | 0.098 (0.185) | 0.113 (0.291) |
Homemaker | 0.107 (0.068) | –0.008 (0.072) |
Mother’s education: | ||
Secondary education | ƒ | ƒ |
Further education | 0.084 (0.064) | 0.168 (0.058)*** |
Higher education | 0.064 (0.069) | 0.219 (0.073)*** |
Father’s status: | ||
Deceased | –0.061 (0.167) | 0.115 (0.173) |
Retired | –0.063 (0.141) | –0.147 (0.142) |
Unemployed | –0.133 (0.171) | –0.016 (0.183) |
Employed full-time | ƒ | ƒ |
Employed part-time | –0.057 (0.138) | 0.131 (0.206) |
Self-employed | –0.027 (0.065) | 0.148 (0.065)** |
Student | 0.331 (0.322) | –0.889 (0.324)*** |
Homemaker | 0.272 (0.324) | –0.235 (0.395) |
Father’s education: | ||
Secondary education | ƒ | ƒ |
Further education | –0.161 (0.063)** | 0.010 (0.067) |
Higher education | 0.006 (0.069) | 0.086 (0.062) |
Examination board | ||
AQAa | ƒ | ƒ |
EDEXCEL | 0.259 (0.083)*** | 0.019 (0.098) |
OCR | 0.198 (0.113)* | 0.295 (0.097)*** |
Other examination board | 0.168 (0.098)* | † |
Other A-levels taken | ||
Mathematics | –0.021 (0.077) | 0.131 (0.082) |
English | –0.095 (0.067) | –0.033 (0.075) |
Physics | 0.021 (0.105) | 0.060 (0.111) |
Statistics and accounting | –0.195 (0.251) | 0.372 (0.194)* |
Science subject | –0.076 (0.075) | 0.001 (0.076) |
Social science subject | 0.149 (0.069)** | 0.224 (0.073)*** |
Humanities subject | 0.261 (0.074)*** | 0.224 (0.072)*** |
Modern languages | –0.139 (0.078)* | –0.217 (0.091)** |
Arts subject | –0.260 (0.142)* | –0.360 (0.091)*** |
Arts subject × male | –0.390 (0.182)** | † |
Estimated threshold parameters | ||
θ1 | 0.621 (0.038)*** | 0.703 (0.043)*** |
θ2 | 1.327 (0.047)*** | 1.454 (0.053)*** |
θ3 | 2.063 (0.054)*** | 2.233 (0.058)*** |
θ4 | 2.824 (0.062)*** | 3.040 (0.067)*** |
Log-likelihood value | –2,943.4187 | –2,767.4346 |
Pseudo-R2 | 0.2004 | 0.2127 |
Number of observations | 2,086 | 2,019 |
Notes:
* significant at 10%, ** significant at 5%, *** significant at 1% levels.
f denotes category omitted in estimation.
† denotes not applicable in estimation.
The standard errors, reported in parentheses, are corrected for heteroscedasticity and for clustering by educational institution.
a AEB and NEAB are grouped together as ‘AQA’ for 1998.
‘Arts subject’ includes Art, Communication Studies, Design and Technology, Graphical Communication, Music, Photography, Theatre Studies and Performing Arts.
‘Humanities Subject’ includes Classical Civilisation, Environmental Studies, Geography, Politics, History, Home Economics, Latin, Law and Religious Studies.
‘Social science subject’ includes Sociology and Psychology.
‘Science subject’ includes Biology, Chemistry, Electronics and Computing.
Table 2 reports the results from the simulations conducted using the two Economics samples. The actual grade distribution for Economics candidates suggests that about 60% of candidates secured a C grade or better in 1998 and in 2000 the comparable figure was close to 63%. A number of simulation exercises are undertaken to establish how the sample of candidates in 2000 would have fared, on average, if they had sat the Economics examination in 1998. Using the 1998 coefficients in conjunction with the 2000 sample, we find that approximately 65% would have achieved a C grade or above, 2 percentage points higher than those who actually achieved these grade allocations in 2000. The average UCAS points score based on the simulation exercise is 6.1 as compared to an actual outcome of 6.0. The grade adjustment required to render the scores comparable is thus 0.1 of a UCAS point. This difference is negligible and is just over one-twentieth of a letter grade. The same conclusion is drawn if we use the 2000 coefficients in conjunction with the 1998 sample. The comparable differential in this case is just over one-tenth of a letter grade. These relatively small differentials (or grade adjustments) could be taken to suggest that standards and/or the ‘relative difficulty’ of the subject have remained constant over the short interval of time reviewed here.
Table 2 Predicted outcomes for 1998 and 2000 Economics samples
A-level grade | Actual Economics outcome in 1998 | Actual Economics outcome in 2000 | Predicted 1998 outcome for 2000 students | Predicted 2000 outcome for 1998 students |
A | 0.226 | 0.248 | 0.276 | 0.190 |
B | 0.175 | 0.183 | 0.187 | 0.173 |
C | 0.191 | 0.196 | 0.186 | 0.199 |
D | 0.165 | 0.166 | 0.150 | 0.182 |
E | 0.110 | 0.110 | 0.095 | 0.128 |
N/U | 0.129 | 0.093 | 0.106 | 0.127 |
Average UCAS points | 5.7 | 6.0 | 6.1 | 5.4 |
Notes:
Predicted Economics outcomes for the 2000 cohort using the estimated coefficients from the ordered probit model for 1998 Economics performance (see equation).
Predicted Economics outcomes for the 1998 cohort using the estimated coefficients from the ordered probit model for 2000 Economics performance (see equation).
Average points scores are based on weighted averages computed using the UCAS points tariff: A = 10, B = 8, C = 6, D = 4, E = 2, N/U = 0.
Conclusion
This paper explored the issue of subject difficulty over a short period of time using an econometric approach. This issue was examined across two years – 1998 and 2000. A quality differential between the two samples in terms of their GCSE background levels was noted, with those taking Economics in 2000 being slightly better qualified than their 1998 counterparts. The analysis confirms the importance of GCSE attainment in determining academic performance in Economics but achievement in GCSE mathematics also exerted an important role.
The primary purpose of this paper has been the implementation of a number of counterfactual exercises using samples of Economics candidates for two separate years. The results of the analysis suggest that the level of difficulty has remained relatively constant over the two years in question. This may be taken to suggest that standards in Economics have been maintained between 1998 and 2000. It should be stressed that we have looked only at one subject over a short two-year time interval. Both cohorts of students took their A-levels before the introduction of Curriculum 2000. An obvious avenue for future research would be to undertake a similar exercise over a longer time period to investigate recently introduced changes involving AS and A-level (and vocational A-level) syllabi. In addition, the methodology used may have a more general application to a review of standards over time in other subjects. Finally, it would be instructive to develop the methodology within a multilevel econometric framework. This requires more detailed multilevel data than are currently available to us, but is clearly worthy of investigation in the future.(note 13)
The Appendix to this paper is in a separate file
Contact details
Ray Bachan
Brighton Business School
University of Brighton
Brighton
Email: rb2@bton.ac.uk
Barry Reilly
School of Social Sciences
University of Sussex
Brighton
Email: b.m.reilly@sussex.ac.uk
References
Ashworth, J. and Evans, L. (1999) ‘Lack of knowledge deters women from studying economics’, Educational Research, vol. 41, no. 2, pp. 209–27.
Ashworth, J. and Evans, L. (2000) ‘Economists are grading students away from the subject’, Educational Studies, vol. 26, no. 4, pp. 475–87.
Cresswell, M. (1996) ‘Defining, setting and maintaining standards in curriculum-embedded examinations: judgement and statistical approaches’, in H. Goldstein and T. Lewis (eds), Assessment: Problems, Developments and Statistical Issues, Chichester: John Wiley and Sons.
Department for Education and Employment (DfEE) (various years) Statistics of Education: Public Examinations, GCSE and GCE in England, London: HMSO.
Fielding A. (1999) ‘Why use arbitrary points scores? Ordered categories in models of educational progress’, Journal of the Royal Statistical Society, A, vol. 162, no. 3, pp. 303–28.
Fielding, A., Yang, M. and Goldstein, H. (2003) ‘Multilevel ordinal models for examination grades’, Statistical Modelling, vol. 3, no. 2, pp. 127–53.
Fitz-Gibbon, C. T. and Vincent, L. (1994) Candidates’ Performance in Public Examination in Mathematics and Science, London: School Curriculum and Assessment Authority.
Fitz-Gibbon, C. T. and Vincent, L. (1997) ‘Difficulties regarding subject difficulties: developing reasonable explanations for observable data’, Oxford Review of Education, vol. 23, no. 3, pp. 291–8.
Goldstein, H. (1995) Multi-Level Statistical Models (2nd edn), London: Edward Arnold.
Goldstein, H. and Cresswell, M. (1996) ‘The comparability of different subjects in public examinations: a theoretical and practical critique’, Oxford Review of Education, vol. 22, no. 4, pp. 435–42.
Greene, W. (2000) Econometric Analysis (4th edn), Upper Saddle River, NJ: Prentice Hall.
Hirschfeld, M., Moore, R. L. and Brown, E. (1995) ‘Exploring the gender gap on the GRE subject test in economics’, Journal of Economic Education, vol. 26, pp. 3–15.
Horvath, J., Beaudin, B. Q. and Wright, S. P. (1992) ‘Persisting in the introductory economics course: an exploration of gender differences’, Journal of Economic Education, vol. 23, pp. 101–8.
LIMDEP 7.0 (1998) Econometric Software, Inc.
MLwiN 1.1 (2000) Centre for Multilevel Modelling, Institute of Education, London.
Oaxaca, R. (1973) ‘Male–female wage differentials in urban labour markets’, International Economic Review, vol. 14, pp. 693–709.
O’Donoghue, C., Thomas, S., Goldstein, H. and Knight, T. (1997) 1996 DfEE Study of Value Added for 16–18-year-olds in England, DfEE Research Series, London: DfEE.
Reilly B. and Bachan, R. (2004) 'A comparison of A-level performance in Economics and Business Studies: How much more difficult is Economics?', forthcoming in Education Economics. Also available at http://www.sussex.ac.uk/Units/economics/dp/reilly85.pdf (Discussion Papers in Economics no. 85, University of Sussex).
STATA 6.0, StatCorp. (1999) Stata Statistical Software: Release 6.0, College Stations, TX: Stata Corporation.
Williams, M. L., Waldauer, C. and Duggal, V. G. (1992) ‘Gender differences in economic knowledge: an extension of the analysis’, Journal of Economic Education, vol. 23, pp. 219–31.
Yang, M. and Woodhouse, G. (2001) ‘Progress from GCSE to A and AS level: institutional and gender differences, and trends over time’, British Educational Research Journal, vol. 27, pp. 245–67.
Notes
[1] We would like to thank Peter Davis, Gwen Coates, Peter Maunder, Walter Heering and participants at the British Educational Research Association annual conference held in Exeter 2002 for providing comments on an earlier draft of this paper. We would also like to thank Min Yang and Antony Fielding for valuable technical advice on multilevel modelling using MULTICAT. We are grateful to Carol Taylor Fitz-Gibbon and Paul Skinner of the CEM Centre, University of Durham, for permission to use ALIS data in this study. The constructive and helpful comments of two anonymous referees are also readily acknowledged. However, the usual disclaimer applies.
[2] A-level examinations in England and Wales are designed for 16–19-year-old students and they act as entry qualifications for university courses.
[3] The UCAS points are: grade A = 10, B = 8, C = 6, D = 4, E = 2, N/U = 0.
[4] These qualifications were replaced by Advance Vocational Qualifications in 2000.
[5] It is not the purpose of this paper to explore subject choice or other potential selectivity bias issues. For instance, the data are selected from ALIS educational institutions and thus may not reflect a random drawing from the population of educational institutions as a whole. This selectivity issue is not one that can be readily addressed and the direction or size of potential bias cannot be known a priori. However, it is recognised as a limitation and some caution should be exercised in regard to the representative nature of the data used and hence the results obtained.
[6] See Fielding (1999) for a discussion of the relative merits of using an ordered model as applied to pupil assessment at Key Stage 1, and Fielding et al (2003) for a multilevel analysis of ordered A-level examination grades as applied to A-level chemistry and geography.
[7] This adjustment relaxes the assumption of observation independence within educational institutions but retains that of independence across institutions. This correction to the variance–covariance matrix is equivalent to adjustments undertaken by educational researchers using multilevel analysis (see Goldstein, 1995). The ordered probit estimates reported in this paper were undertaken using the LIMDEP 7.0 and STATA 6.0 software packages.
[8] The approach is based on the ‘index number’ approach popularised in labour economics (see Oaxaca, 1973). It is thus subject to a conventional ‘index number’ problem where the result obtained is sensitive to the coefficient vector used. Reilly and Bachan (2004) provide further details of the methodology as used in the ordered probit case.
[9]
The separation of the samples by year is justified on the basis of likelihood ratio tests. The null hypothesis of common ordered probit parameters across the two years against the alternative of different parameters for each year is rejected by the data with a = 75.1 . The null hypothesis incorporating an intercept shift for one of the two years is also rejected against the same alternative with a
= 73.1 . The chi-squared values can be transformed into z-scores based on the fact that
~ N(
, 1) for large k. The corresponding z-scores for 1998 and 2000 are 2.6 and 2.5 respectively and these comfortably exceed the ±1.96 critical values at the 0.05 level.
[10] We recognise that there is a potential correlation in unobservables across the two years that act through the educational institutions. The failure to deal with this might have implications for the coefficient estimates and their standard errors.
[11] The marginal effects are not included to conserve space, but are available from the authors on request.
[12] The gender effect in performance for 2000 is not directly interpretable from the gender coefficient in the performance equation given the use of a gender interaction with the continuous GCSE score. The average effect on performance of being male in 2000 (using the average GCSE score from Table A1) is given as 1.425 – 0.177 × 6.31 = 0.31. In rendering a comparison across years, it is also worth noting that the 1998 gender coefficient is an average male effect for those who did not take an arts subject at A-level and those not in a grant-maintained school.
[13] An ordered logit fixed-effects model incorporating the two levels available to us (individuals and institutions) was also estimated using the MULTICAT macro provided by the Centre for Multilevel Modelling, Institute of Education, University of London, for use with the MLwiN1.1 software package. The substantive findings reported in Table 2 were not materially altered in using the ordered logit two-level coefficients. Although this is a heartening result, it remains to be seen whether such results would hold with the use of deeper-level information.