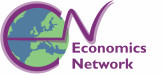
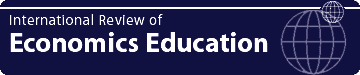
Measuring and Responding to Variation in Aspects of Students’ Economic Conceptions and Learning Engagement in Economics
Martin P. Shanahan and Jan H. F. Meyer1
International Review of Economics Education, volume 1, issue 1 (2003), pp. 9-35
DOI: 10.1016/S1477-3880(15)30209-7 (Note that this link takes you to the Elsevier version of this paper)
Up: Home > Lecturer Resources > IREE > Volume 1 Issue 1
Abstract
Meyer and Shanahan (1999) introduced an embryonic model of student learning in economics based on an initial consideration of various forms of school-leaving prior knowledge, including (a) subject-specific prior knowledge, (b) conceptions of learning and (c) preferential or habitual forms of learning engagement. Results across two universities (n > 1,300) confirmed the statistically significant effects of having studied economics at school, the status of English as a second language, and basic economic misconceptions, on learning outcomes at the end of semester one.
A cluster analysis approach to separating out subgroups of students exhibiting differential patterns of association between economic misconceptions – essentially pathological process components of learning engagement – and learning outcomes was reported. It was concluded that the various forms of prior knowledge considered represented a valid foundation for the construction of a more complex model of student learning specific to the subject of economics.
The present study reports on the subsequent analysis of aspects of a more complex model of student learning specific to economics. In particular, longitudinal measures of one dimension of students’ prior knowledge and students’ prior economic conceptions are presented, using student responses from one university (initial n > 680). Institutional responses are presented that emerged as a consequence of the systematic identification of students ‘at risk’ of failing, and the theoretical underpinning of such responses, and comments on their impact on students’ prior knowledge.
Earlier pilot work (Meyer and Shanahan 2001a) suggested that limited intervention to raise students’ meta-learning capacity over one semester has a comparatively neutral impact. But viewed as a whole, findings thus far indicate that targeted teaching interventions that respond to individual variation in learning engagement may have a positive impact on learning outcomes. The impact of such interventions on students’ prior knowledge of economic conceptions is less clear. That such interventions are practically possible as well as theoretically justified is the subject of further research.
JEL Classification: A22
Introduction
Students frequently find the transition between high school and university studying difficult. The subject of economics is perceived by many students as particularly difficult, despite its importance as a fundamental subject of study for business, accounting and commerce students. One reason for this difficulty is students’ habitual or preferential approaches to learning engagement on entry to university.
The present paper builds on earlier work focusing on entering first-year students of economics at the universities of South Australia and Adelaide (Meyer and Shanahan 1999, 2001b; Cowie et al., 1997). This previous work demonstrated the capacity of an economics-specific model of student learning to identify entering first-year students who are potentially ‘at risk’, in the sense of being unlikely to cope with the subsequent demands of the first year. This modelling approach recognises that factors contributing to many of the problems that first-year students experience stem from their prior experiences of, and approaches to, learning engagement. Simply put, the learning history2 of a student represents a source of explanatory variation that has predictive power in modelling learning outcomes.
On entry to university in particular, it is possible to capture aspects of students’ learning histories in a manner that can inform judgements about the likelihood of certain students being unable to cope with the looming demands of university study in the absence of any supportive interventions. Learning history data can also be used by institutions to shape their response to problematic patterns of variation in student learning. Students are generally willing to provide such information if they are given assurances that such informed disclosure is intended to help them as individuals and inform teaching practice.
An initial economics-specific model of student learning revealed that subject-specific prior knowledge, conceptions of learning and learning history impacted significantly on student learning engagement and learning outcomes (Meyer and Shanahan, 1999, 2001a, 2001b). Important explanatory factors that were identified included whether a student held economic misconceptions, their view on what constituted ‘economic activity’, whether English was a second language, their prior level of mathematical study, plus a number of additional observables related to their conceptions of learning, after the work by Meyer and Boulton-Lewis (1999).3
The model was thus consistent with the view that entering first-year university students bring with them, as part of their learning history, varying forms of explanatory prior knowledge. Some of this prior knowledge may be grounded in subject-specific terms: for example, what students ‘know’, or believe to be ‘true’, about aspects of a subject such as economics. A different form of prior knowledge refers to students’ beliefs about ‘knowledge’ and conceptions of ‘learning’. In modelling terms, these two forms of prior knowledge, together with other generic sources of variation such as learning intention, motivation and process, partially explained what was considered to be an educationally significant proportion of the variation that students consequently exhibited in their contextualised learning engagement in economics, as well as in resultant learning outcomes.
The specific model was also consistent with a more general learning model that posits the concept of dissonance as important in placing students ‘at risk’ (Meyer and Vermunt, 2000). In general terms, dissonance in learning engagement arises when students’ preferred patterns of learning engagement are in conflict with the learning environment and its demands. Resultant manifestations of learning engagement are typically characterised by a failure to distinguish between aspects of learning and perceptions of the learning environment that are basically incompatible. Students who are unable to adjust dissonant patterns of learning engagement to the unfamiliar and possibly even hostile demands of a changing (or changed) learning environment, as typically experienced in the transition from school- to university-based studying, are clearly academically ‘at risk’.
In general, the dynamics of learning engagement in the first year of university study, and the attendant ‘risk’ attached thereto in terms of dissonance, is well established, and Meyer (2000a) has proposed a conceptual framework for formalising this phenomenon. Patterns of stable ‘at risk’, and of deteriorating, learning engagement (relative to entry level) have been clearly associated with academic failure or low achievement in a number of individual-difference studies. This same issue is revisited here in the context of the economics-specific learning model with the aim of developing a conceptually sound and sensitive response to students potentially ‘at risk’. This is an important and socially relevant goal that is crucially dependent on both the diagnostic attributes and dynamics of the underlying model that is being developed.
Associations between contextualised learning engagement and learning outcomes usually occur in a temporal sense: that is, the period between a student’s disclosure of their learning engagement and the learning outcome (examination results) is relatively brief. In contrast, our earlier work has modelled the relationship between entry-level aspects of learning engagement and learning outcomes over a period of one semester (approximately 4 months) on the perhaps unreasonable assumption that the explanatory sources of variation were relatively stable.
The present study reports on a further stage of the development of an inferential model of student learning, aspects of which are subject-specific to economics. Of particular importance are the dynamics of learning engagement and the longitudinal stability of explanatory forms of discipline-specific prior knowledge: that is, learning outcomes are being directly modelled here in terms of key aspects of students’ beliefs about economic phenomena.
The remainder of the present study is in four sections. The first provides an overview of the results from previous modelling and outlines the institutional structures and responses that form the background of the study. The second provides details about the data used (and omitted) and comments on the implications of these for the interpretation of the model. The third section reports on the stability (or otherwise) of some of the economics-specific forms of prior knowledge observables over the course of one semester. The final section discusses the implications of these findings for the economics-specific model, and comments on the effectiveness of current institutional interventions.
Results from previous modelling
Meyer (1999) and Meyer and Shanahan (1999) have previously reported on the conceptual framework underpinning the economics-specific learning model. Interested readers are directed to these papers and the further calibrations of the observables reported in Shanahan and Meyer (2001) and Meyer and Shanahan (2001a, 2001b). For the purposes of providing background to the present study, the modelling outcomes are briefly summarised here.
In 1998 entering first-year students at two Australian universities (the University of South Australia, n = 689; and the University of Adelaide, n =448) completed an economics-specific learning inventory. Resultant analyses (Meyer and Shanahan 1999) isolated three separate subject-specific aspects of prior knowledge whose corresponding subscales were psychometrically robust: an economics misconceptions subscale (EMC), an economist activity subscale (EAC) and an economic reasoning subscale (ERE). A fourth dimension, labelled ‘economic worth’ (capturing variation in students’ conceptions of price determination), followed essentially from the phenomenographic study by Dahlgren (1984). Contrasting aspects of this ‘worth’ dimension were subsequently operationalised via two subscales, the first locating students’ conceptions that prices were fundamentally an outcome of market forces (MKT), and the second identifying conceptions that price reflected the intrinsic worth of a good (INT). Meyer and Shanahan (2002) present a full discussion of the psychometric development of these latter two subscales, which are integral to the present study.
These subject-specific prior-knowledge subscales were incorporated into an existing (Likert-type response format) inventory of student learning, containing established modelling process observables. In completing this inventory, students were asked if they had studied economics as a school subject. The responses to this question (yes or no) defined a further categorical observable (of subject-specific prior knowledge) in the subsequent analyses. A second categorical (yes or no) observable, which was not subject-specific, captured the ESL (English as a second language) status of students. Further aspects of students’ prior knowledge (of learning conceptions; what learning ‘is’) were surveyed based on a subset of the observables imbedded in the Reflections of Learning Inventory: RoLI (Meyer and Boulton-Lewis, 1999). This inventory, now in the final stages of development, operationalises conceptions of learning that traverse an accumulative–transformative emphasis of beliefs about what ‘learning’ is. (For some students learning is about collecting facts and information; for others it is about understanding new material, possibly seeing things in a different light as a consequence, and even changing as a person.) The inventory also incorporates some observables that emerged as being of particular interest in the modelling of student learning in economics: (a) an external learning motivation expressed in terms of a moral obligation or duty (DUT), (b) an epistemological belief that knowledge is basically discrete and factual (KDF), (c) ‘memorising’ before ‘understanding’ (MBU) and (d) ‘memorising’ as a process of rehearsal (MAR). The conceptual distinction between these and other contrasting forms of ‘memorising’ and their psychometric operationalisation is fully dealt with by Meyer (2000b, 2000c).
Comparisons of student responses between the two universities revealed that, regardless of the institution, students who had not studied economics at school exhibited a higher level of economic misconceptions (a statistically significant higher median score) than those who had studied economics previously, while students who had not studied economics at school had a statistically significant lower median score on the subscale ‘economic activity’ than those who had studied economics previously. An analysis of end-of-semester examination results (derived from essentially the same examination at both universities) also revealed that, in every part of a three-part examination (identified further on as Parts A, B and C), students for whom English was a second language did significantly worse than students for whom English was a first language. Parallel results were found for students who held economic misconceptions at the beginning of the semester. These findings, consistent across two dissimilar institutions, were considered to be quite remarkable given the ‘non-temporal’, one-semester interval between the gathering of the learning engagement history data and the learning outcome measures.
Having noted these effects of prior knowledge on examination outcomes, the research was broadened to examine underlying multivariate associations between the explanatory observables and learning outcomes via exploratory factor analysis. Four conceptually interpretable dimensions of variation – a ‘conceptions of learning’ factor, a ‘learning pathology’ factor, a ‘deep-level processing’ factor and a ‘learning outcomes’ factor – were identified.
Surprisingly, there was no evidence of a positive association in the factor structure between the outcome measures and any of the deep-level learning processes represented, in particular, by ‘use of evidence’ and ‘relating ideas’. The ‘learning outcomes’ factor, however, exhibited conceptually interpretable negative associations between all three components of the final examination on the one hand and economic misconceptions (EMC), as well as with (to a lesser degree) motivation expressed as discharging a duty (DUT), memorising before understanding (MBU), an epistemological belief that knowledge is discrete and factual (KDF), together with the observables that separately also loaded on the pathological factor. The substantive composition of this fourth factor, representing the only dimension of variation that clearly linked learning outcomes with some of the explanatory (independent) observables representing especially learning pathologies, was retained for further exploratory modelling purposes. And it was recognised that what was being modelled was academic failure or low achievement rather than academic success.
This recognition is congruent with an important motivation for the present work: namely, the identification and support of students ‘at risk’ of failure. This focus makes the preservation of the observed individual response, rather than a statistical abstraction of it (such as a factor score), important. The modelling approach adopted was therefore conservative and was intended to identify those first-year students who, on entry to university study, exhibit patterns of learning engagement that are likely to be at variance with what the course expects of them.
Consistent with this aim was an earlier study that categorised individual-similarity subgroups according to the degree that learning pathologies ‘interfered’ with otherwise sound (‘deep-level’) process patterns of learning engagement that are conceptually and empirically normally associated with learning outcomes that reflect the acquisition of understanding. In a previous General Linear Model these process categorisations and other entry-level data explained some 46 per cent of the variation in subsequent end-of-semester examination performance (Cowie et al., 1997). Learning pathologies thus played an important modelling role in this earlier work; a role that was consistent with the results of the above explanatory factor analysis in respect of the composition of the ‘learning outcomes’ factor.
In thus selecting the defining features of the ‘learning outcomes’ factor as the basis of a more conservative modelling approach, it was emphasised that what was retained was, at best, an embryonic and partial model. The partial nature of the model arises because only one of the four empirically identified dimensions of variation suggested by the earlier factor analysis empirically justified further exploration. Furthermore, this partial model was tied to a specific response context (University of South Australia, 1998 first-year cohort) and its validity was not advocated beyond this possibly unique context.
Using k-means clustering, a simple two-cluster solution exhibited the expected inverse theoretical linkages between (a) low mean scores on the explanatory observables and high outcome (exam) mean scores in one cluster and (b) high mean scores on the explanatory observables and low outcome (exam) mean scores in the other cluster. An ideal expectation was that a finer partitioning (extracting more clusters) would result in a solution in which the defining modelling features of the two-cluster solution would not be compromised but reproduced in finer detail in terms of the differences in mean score patterns for each cluster.
The decision of how many clusters to extract was driven not by statistical criteria but by a pragmatic expectation based on comparable individual-difference studies that, in a large heterogeneous first-year sample, between 10 and 15 per cent of the students would exhibit some form of ‘high-risk’ learning behaviour associated with poor academic performance. Using this expectation as a guide, a finer-grained five-cluster solution was also examined (see Figure 1), in which Cluster 4 (the ‘high-risk’ cluster; n = 90; some 13 per cent of the sample) and Cluster 5 (the ‘low-risk’ cluster; n = 175, about 26 per cent) generally preserved the features of the two-cluster solution. Notwithstanding the variation within these two subgroups, there emerged modelling information that was again consistent with theoretical expectations. There was also encouraging evidence that the intermediate subgroups (clusters) generally exhibited patterns of association that were also in accordance with theoretical expectations, but only so in respect of the Part A and Part B examination outcomes. And while the finer-grained clusters did result in some apparent anomalies, these could be plausibly explained.
As interesting as these results were, however, they only utilised student responses from a learning inventory distributed at the beginning of the semester against end-of-semester examination results. One unanswered question concerned the intermediate dynamics of learning engagement. Indeed, the modelling approach thus far had assumed that the entry-level learning aspects of learning engagement and forms of prior knowledge remained stable during the first semester – a period of several months. Previous studies have demonstrated that patterns of stable ‘at-risk’, as well as deteriorating, learning engagement (relative to entry level), are associated with academic failure or low achievement. To this point, the economics-specific modelling had not addressed, in particular, how the forms of economic prior knowledge were impacted upon by institutional responses designed to raise students’ meta-learning capacity. This question is the focus of the remainder of the present study.
The institutional context and response initiatives
The institutional responses referred to above need to be placed in context. The University of South Australia is a comparatively new university formed from the amalgamation (in 1991) of the South Australian Colleges of Advanced Education and the South Australian Institute of Technology. It has approximately 25,000 students and 2,000 staff over six campuses. The university attracts students from diverse educational and cultural backgrounds and has a stated aim to ensure equity and access in both its intake processes and the assistance it provides students. Many students are the first in their family to attend a university.
The Division of Business and Enterprise teaches approximately 25 per cent of the university’s students. It offers programmes in information technology, marketing, accounting, management, logistics, commercial law and international business. Within the first year of a 3- or 4-year degree, all students study a ‘core’ of courses considered fundamental to business studies. One of these is ‘Economic Environment’ – essentially an introduction to macroeconomics. It is the responses of students enrolled in the ‘Economic Environment’ course (hereinafter simply referred to as ‘the course’) in the first semester of 2001 that are considered further on.
The institutional context that frames the present work articulates with the University of South Australia’s policy to improve access to university for non-traditional entrants. Such an intention carries with it a commitment by the institution to develop mechanisms that can assist in the early identification, and subsequent supportive management, of students who are potentially ‘at risk’ of failure or low achievement on entry to university by virtue of preferential or habitual modes of learning engagement, and forms of prior knowledge, that are inappropriate to university study. At the university level, this has meant a commitment to funding teaching and learning initiatives at the discipline, division and institutional levels.
In 2001 several institutional initiatives were introduced into the course with a view to responding positively and appropriately to variation in student learning. Underlying each particular initiative was the objective of embedding an awareness of individual variation in student learning into the teaching environment. Such change, however, was contained by an external budgetary constraint that meant that any change had to be sustainable within existing resources.
The most important institutional step was the incorporation of an explicit emphasis on ‘learning to learn’ within the course itself. This placed learning issues within the discipline of economics, rather than positioning them in some way ‘outside’ or ‘peripheral’ to the course. The result was that, in addition to presenting material on first-year macroeconomics, the course also contained material on ‘learning to learn’. For the students, there were several ‘outward’ signs of this change in emphasis.
The first sign of this change was the incorporation of the student inventory on to the home web-page of the course. This placed the measuring instrument within the framework of the course itself. Second, a student who completed and submitted a response to the inventory was awarded a bonus 2 per cent towards their final grade (4 per cent in total when they completed the first and second inventories). This step not only increased the proportion of student responses to the inventory, but also enhanced the legitimacy of the exercise in the minds of students, who perceived the emphasis on learning issues to be part of the course, rather than external to it.
In addition to including the measuring instrument within the course, exercises were incorporated into the weekly workshops that were aimed at raising general student awareness of learning issues. These exercises, loosely based on the concept of developing students’ meta-learning capacity after the work of Biggs (1985), were aimed at raising students’ awareness of variation in what ‘learning’ could mean: variation in how students (including themselves) could, and did, go about ‘learning’ something, the institutional expectations and interpretation of what ‘having learnt’ something meant, and variation in methods of assessing learning. Several exercises involved students ‘linking’ learning issues with learning techniques and assessment techniques. Each set of exercises required approximately 10 minutes out of a 45-minute class, as well as requiring students to prepare outside the class. These exercises were not formally assessed but aimed at increasing students’ awareness of meta-learning issues. It was also assumed that exposure to the course content would influence later measures of students’ ‘prior’ economic knowledge.
The six tutoring staff consisted of postgraduate students, with one casual tutor. Although one had qualifications as a schoolteacher, the remainder consisted of economics graduates without formal educational training. These staff, together with the two lecturers in the course, attended 3 days of workshops by the second author (Meyer). These workshops, with antecedents in the core module of the Postgraduate Certificate in Higher Education taught at the University of Durham, introduced all the staff to the theory and practical consequences that result from taking variation in student learning seriously from a teaching perspective.
Midway through the semester (week 7 in the 13-week semester), all students were contacted individually and invited, should they so wish, to discuss learning issues with their tutors. There was no compulsion in the invitation and it was not linked to assessment. This formal ‘invitation’ was additional to the standard weekly invitation that students were routinely given by tutors to contact them should they require assistance. Further, there already existed a ‘help desk’ aimed at assisting students with economic concepts at times when tutors and other staff were unavailable. The role of this facility was broadened to include ‘learning issues’ and it was staffed by lecturers who had attended the learning workshops.
The data
There are three components to the data used in the present study. In semester 1, 2001, students enrolling in the course (total n = 688) were asked to complete a web-based student-learning inventory and to do so within the first 3 weeks of the course. This inventory comprised 105 items responded to via a Likert-type format (with responses scaled 1 to 5) and containing economics-specific prior knowledge items together with established modelling process observables. Students were asked to contextualise their responses in terms of their most recent school experiences of studying economics or a cognate subject.
In completing the inventory, students were additionally asked their gender, if they had studied economics as a school subject, whether English was a first or second language, whether they completed their education in a public (state-funded) or private school, whether their school was single sex, whether an immediate family member had attended university, and whether they enjoyed maths, hobbies or sport. Some of the responses to these questions permitted the definition of additional categorical observables (of subject-specific prior knowledge) as well as non-subject-specific observables (such as English-language status).
The second component of the data consists of students’ responses (total n = 483) to a second, virtually identical learning inventory completed between weeks 11 and 13 (the final teaching week). A crucial difference between the first and second inventory, however, is that students were asked to respond to items in the context of their first-semester university experience of studying economics in the course.
Offering students ‘bonus’ marks towards their final assessment if they completed the inventories was designed to encourage their participation, which was not compulsory. The difference in student numbers completing the first and second inventories (688 versus 483), therefore, is not just caused by students withdrawing from the course, but it also reflects varying degrees of willingness to participate. There is the additional complication that some students completed the second but not the first inventory.
The third component of the available data set consists of students’ examination marks. Students sat a 2½-hour examination 1 week after the end of the semester (total n = 538). The examination consisted of three parts, each of equal weight. Part A involved 25 multiple-choice questions (five options per question) and was aimed at assessing fundamental concepts and definitions. Part B involved selecting one of two short-answer questions that were based on an accompanying newspaper article, and responding with answers that were framed by given economic models. This section of the paper was aimed at assessing the application of knowledge within a given framework. Part C of the paper involved a single compulsory essay and was designed to allow students to demonstrate their knowledge in a comparatively unrestricted way. Each part of the examination was recorded separately.
As in our earlier work on seeking to explain variation in learning outcomes, there is an assumption that the outcome measures are valid representations of qualitatively different forms of ‘learning’: ‘factual understanding’ (Part A), ‘application’ (Part B) and ‘deeper understanding’ (Part C). The learning outcomes were strictly retained in the form in which they were observed because they capture the ‘academic reality’ of the completed examination process.
The construction of the data provides a number of possible insights, of which only one is explored here. Of particular interest is the stability of students’ measured prior knowledge, and especially their prior economic conceptions, as measured using selected inventory subscales, and whether any changes in these observables are in line with theoretical expectations and associated with end-of-semester outcomes. (Brief explanations of the economic prior-knowledge inventory subscales are presented in Appendix 1).
It is emphasised that, in this next step of modelling outcomes directly in terms of prior knowledge, consideration is not being given here to other available sources of explanatory variation, such as learning processes, motivations or intentions. The aim here, in fact, is specifically first to establish the explanatory power of discipline-specific aspects of prior knowledge. This aim is foregrounded against the relatively few studies that have attempted to model learning outcomes exclusively on this basis (some attempts have been made in terms of conceptions of learning) and there are no comparable studies that the authors are aware of that have attempted to do so in discipline-specific terms.
Results
A total of 446 students completed both the first and second inventories and, of these, 405 also completed the final end-of-semester examination. It is clear that the available data set is biased in that it does not include all students who sat the course and completed the exam. It is not obvious, however, whether there is any systematic bias in the sample, as initial inspection of those students omitted from the analysis does not reveal any clear trends in student type, gender, approach to studying or outcomes. It is nevertheless possible that our findings are not truly representative of the student body as a whole, and investigation into sample bias is continuing. Our results should be interpreted accordingly.
An initial inspection of the changes in learning observables across the semester, using a paired-samples t-test, reveals that, of the 20 observables, 10 exhibit a statistically significant change in mean value: EMC (economic misconceptions, p < 0.001), MAR (memory as rehearsal, p < 0.001), SDI (seeing things differently, p = 0.002), MBU (memorising before understanding, p = 0.002), RID (relating ideas, p = 0.001), KDF (seeing knowledge as discrete and factual, p = 0.043), DRP (detail-related learning pathology, p < 0.001), MWU (memorising with understanding, p = 0.017) and FRA (fragmentation, another learning pathology, p < 0.001). Table 1 provides full details of the results for all the observables.
One problem with such an analysis is that while it provides information about the significance of differences in means at the group level, it provides no insight into possible differential effects at a selected subgroup level that might be of particular interest. Second, as the range of possible distributions within each scale ranges from 5 to 25 (five items per subscale with responses ranging from 1 to 5), and depending on the actual distribution of student responses, the differences in mean values may be quite small and still significant.
Table 1 Comparison of the mean scores on the first and second inventories (paired samples) of first-year economics students, 2001
Observable | Mean value 1st inventory | Mean value 2nd inventory | Mean difference | 95% confidence interval of the difference | t-score | p-value (two-tailed) | |
Lower | Upper | ||||||
EMC | 12.86 | 12.29 | 0.57 | 0.27 | 0.86 | 3.74 | <0.001 |
EAC | 18.33 | 18.15 | 0.17 | –0.10 | 0.44 | 1.26 | 0.208 |
ERE | 17.21 | 17.36 | –0.15 | –0.40 | 0.11 | –1.14 | 0.253 |
MKT | 18.49 | 18.62 | –0.12 | –0.42 | 0.17 | –0.83 | 0.407 |
INT | 14.12 | 14.29 | –0.17 | –0.54 | 0.21 | –0.88 | 0.381 |
MAR | 16.84 | 17.54 | –0.69 | –1.01 | –0.37 | –4.27 | <0.001 |
FAC | 17.07 | 16.99 | 0.08 | –0.21 | 0.37 | 0.53 | 0.597 |
IND | 19.95 | 19.98 | –0.03 | –0.28 | 0.22 | –0.23 | 0.818 |
SDI | 20.51 | 20.09 | 0.42 | 0.15 | 0.69 | 3.10 | 0.002 |
MBU | 15.07 | 15.61 | –0.54 | –0.88 | –0.19 | –3.08 | 0.002 |
RID | 19.65 | 19.23 | 0.42 | 0.18 | 0.66 | 3.38 | 0.001 |
KDF | 14.02 | 14.35 | –0.32 | –0.64 | –0.01 | –2.03 | 0.043 |
MAU | 18.30 | 18.35 | –0.04 | –0.30 | 0.22 | –0.30 | 0.764 |
DRP | 14.16 | 15.13 | –0.97 | –1.25 | –0.70 | –6.89 | <0.001 |
RER | 19.06 | 19.05 | 0.01 | –0.23 | 0.26 | 0.11 | 0.914 |
DUT | 13.73 | 14.03 | –0.30 | –0.61 | 0.01 | –1.91 | 0.057 |
MWU | 19.64 | 19.35 | 0.29 | 0.51 | 0.52 | 2.39 | 0.017 |
FRA | 12.97 | 13.97 | –1.00 | –1.30 | –0.70 | –6.58 | <0.001 |
RAU | 19.07 | 18.99 | –0.08 | –0.21 | 0.38 | 0.55 | 0.584 |
LBE | 15.31 | 15.58 | –0.27 | –0.57 | 0.04 | –1.71 | 0.088 |
Source: University of South Australia, first-year students, 2001.
Notes: n = 446, Df = 445. All values (except p-values) rounded to two decimal places. EMC (holds economic misconceptions); EAC (economic activity scale); ERE (economic reasoning); MKT (views prices as set by market or intrinsic value); INT (views prices as reflecting intrinsic value); MAR (memorises as rehearsal); FAC (conceives of knowledge as facts); IND (thinks independently); SDI (views knowledge as seeing things differently); MBU (memorises before understanding); RID (views knowledge as relating ideas); KDF (conceives of knowledge as discrete and factual); MAU (memorises as an aid to understanding); DRP (detail-related pathology); RER (rereading a text); DUT (motivated by extrinsic sense of duty); MWU (memorises with understanding); FRA (fragmentation pathology); RAU (repetition as an aid to understanding); LBE (learning by example). The t-test statistic provides information about whether the null hypothesis can be rejected at a predetermined level of confidence (in this case 95%), while the p-value provides an exact measure (to three decimal places) of the probability of committing a Type I error.
A more insightful approach that allows for the identification of subsets of students within the data exhibiting differential effects utilises k-means cluster analysis. A k-means cluster analysis results in k distinct clusters being formed using an algorithm that minimises variability within, and maximises variability between, the clusters. This process may be thought of as a ‘reverse form’ of analysis of variance. It is used here to exhibit individual differences at a subgroup (cluster) level based on a direct consideration of the relationship between what students have disclosed about themselves and learning outcomes. The interest in individual differences emanates from an endeavour to construct insights into how students potentially ‘at risk’ of failure in the first-year transition period may be detected in a short space of time and on a relatively large scale. In general, this endeavour requires a methodology that can preserve individual student responses as ‘real people’ within the analytical process rather than (as noted earlier) statistical abstractions via, for example, factor score estimates. The focus here is specifically on the economic observables EMC, MKT and INT, and it transpires that these three observables exhibit conceptually powerful modelling power.
In terms of EMC (economic misconceptions) alone, as Figure 2 confirms, those students who on average are located in the cluster that recorded low levels of economic misconceptions (Cluster 7, n = 49) performed best in Parts A and C of the final exam and second best in Part B of the exam. As a subgroup these students in Cluster 7 exhibited the lowest levels of EMC early in the course (EMC1), which decreased further over time (EMC2). (In Figure 2 and subsequent figures, the suffix 1 identifies an observable in the first inventory by week 3 while the suffix 2 does so in the second inventory between weeks 11 and 13). At the other extreme, students located in Cluster 5 (n = 44), who recorded the highest and increased levels of economic misconceptions, were also unambiguously worst on all parts of the final examination. There is an unmistakable impression here, in terms of understanding some basic economic phenomena, of the poor getting poorer and the rich getting richer, with theoretically expected outcomes in each case. Thus in asking students about their ‘economic misconceptions’ before the end of week 3, this essentially univariate-predictor cluster model suggests it is possible to identify early on some students who will, on average, perform best and worst on all sections of the final exam. Even taken on its own, such an insight, so easily interpreted, has the potential to provide lecturers with an important ‘early warning’ signal as to which students may be potentially ‘at risk’ as well as those relatively less so.
The observation that those students who collectively recorded the lowest economic misconceptions by week 3 of the semester recorded still lower misconceptions in week 13, while those who recorded serious levels of misconceptions in week 3 actually did worse in week 13, deserves further comment. One plausible inference from such a result is the suggestion that other factors, such as learning processes (not analysed here) may also be strongly associated with measured economic misconceptions. In particular, it seems reasonable to suggest that those students exhibiting low levels of economic misconceptions may also exhibit low scores on ‘negative’ or detrimental learning processes compared to those students who on entry exhibit high levels of economic misconceptions. While such analysis is the basis of ongoing research, another alternative conjecture – that holding high levels of economic misconceptions may also be related to the level of prior exposure to economics – is addressed later.
Within the extremes of Clusters 5 and 7 depicted in Figure 2, there is an interesting mix of inferred relationships. The trajectory (cluster-means plot) of students represented by Cluster 6 (n = 59) membership clearly parallels those in Cluster 5, albeit in a less extreme manner, while students in Cluster 4 (n = 72) seem to have gone ‘against the trend’. More worrying is the trajectory of students in Cluster 1 (n = 31) who, while performing comparatively well on the measures of economic misconceptions, performed second worst on examination outcomes. On the other hand, students in Cluster 2 (n = 66), while falling in the ‘mid-range’ of students, do comparatively well on the separate parts of the final examination. These latter two theoretically problematic findings are a strong reminder that a single ‘economic misconceptions’ subscale on its own is insufficient to identify ‘at-risk’ students, although its ability to identify (as a source) the extremes among students is highly tantalising for those seeking a simple and short method of identifying subgroups of students potentially ‘at risk’. The reality is that such an observable only estimates one aspect of student prior knowledge and reveals nothing about learning engagement in process terms. It is thus perfectly feasible that students who, for whatever reason, exhibit comparatively low levels of economic misconceptions may also exhibit pathological forms of learning engagement that are detrimental to overall examination success, while those with more modest misconceptions also possess comparatively advantageous approaches to learning.
Figure 3a addresses some of these issues by presenting the modelling power of three observables, MKT (viewing price determination as primarily the result of the market), INT (viewing price determination as primarily reflecting intrinsic worth) and EMC (economic misconceptions), against Parts A, B and C of the final exam. Conceptually, the MKT and INT scales are essentially negatively related to the extent that a student who holds the conception that markets are the primary determinant of prices is unlikely to concurrently hold the conception that the intrinsic worth of a good determines its price.
Figure 3a again reveals that students in Cluster 5 (n = 52) scoring the highest on economic misconceptions, and who strongly view prices as the result of intrinsic worth (INT), and who are in the mid-range on price determination (MKT), unambiguously do worse on all sections of the final examination than students in other clusters. This observation is in complete accord with theoretical expectations. The suggestive inference is that of a group of students who, on entry to the university, and throughout the semester, conserve forms of economic prior knowledge that are ultimately detrimental to their performance in an end-of-semester economics examination. Moreover, this appears to be so despite formal teaching implicitly intended to erase such misconceptions.
Another illustration of the consistent modelling power of MKT, INT and EMC lies in their apparent capacity to identify another opposite extreme in learning outcomes (Cluster 4; n = 50). By comparison, Cluster 7 (n = 80) identifies a group of students who scored in the ‘mid-range’ on these measures and also scored in the ‘mid-range’ on all three parts of the final examination. Again, these findings are consistent with theoretical expectations. In similar vein, Cluster 1 (n = 62) and Cluster 3 (n = 56) appear (in terms of the prior-knowledge observables) to separate out two otherwise quite similar subgroups of students who perform quite differently in respect of the multiple-choice Part A of the examination, versus Parts B and C. The question of what additional background factors may explain this separation remains open.
Interestingly, students with a relatively low level of economic misconceptions, and who also score comparatively low on a scale measuring conceptions that prices result from a good’s intrinsic worth, but who also score lowest in viewing markets as the primary determinant of price, do second worse in the final examination (Cluster 6, n = 43). These students, it would appear, bring with them to the course a comparative lack of knowledge about the link between markets and prices, even if their views on worth and price seem relatively certain. Another group of students whose responses are suggestive of the importance of students’ prior knowledge of market and prices is captured in Cluster 2. These 62 students, despite scoring comparatively highly on economic misconceptions, and who ranked equal highest on the INT scale and highest on the MKT scale, performed second best (on average) across all three sections of the exam. The suggestion is that students who bring with them (and retain) strong conceptions about the link between markets and price do relatively well, and here the earlier comment on the importance of considering more than a single dimension of variation in students’ prior learning and learning engagement again seems appropriate.
Figure 3a also highlights the comparative stability, even within different subsets of the total student body, of prior economic conceptions. For example, in almost every cluster, there is virtually no change in mean score on the scale measuring students’ conceptions of the relation between price and market forces. While greater change across the semester is evident for the INT and EMC observables, there is no evidence of a dramatic shift from say, high levels of economic misconceptions on entry to university and end-of-semester conceptions (as measured by these scales). Such a finding is suggestive of the persistence of deeply held prior knowledge and that one semester’s exposure to economics may be insufficient to alter such prior conceptions. This observation confirms a similar conclusion reached in an earlier study (Meyer and Shanahan, 2001a).
Figures 3b and 3c respectively present results from an exploratory partitioning of the data into students who studied economics at school (n = 179) and students with no prior (school) knowledge of economics (n = 226). This partitioning seeks to address the issue, raised earlier, that economic misconceptions (EMC) are related to a student’s prior exposure to economics. Earlier work (Meyer and Shanahan, 1999) did report such a connection. The aim here is to investigate this relationship further by introducing the two additional observables related to price determination (INT and MKT).
Figure 3b reveals more variation in, and separation between, the cluster means for INT between inventory 1 and inventory 2 than is exhibited in Figure 3a. Also somewhat comforting is that, with the exception of Clusters 2 and 7 (total n = 57), all other clusters exhibit a decrease in the mean score on economic misconceptions across the semester. Less comforting, however, is the apparently high degree of variation between the mean scores on the first and second INT scale, with movements in both directions. Thus among students who have previously studied economics, another semester of economics does appear to impact on their measured views on the (proximal) association between price and intrinsic worth – although not always in a direction that would be considered desirable.
Also, of the students who had studied economics previously, Cluster 1 (n = 17), the group with the largest apparent improvement in economic misconceptions (which were not high initially) and the lowest mean score on INT, and who had the largest drop in this score, did not do particularly well on Parts B or C of the final exam. Cluster 4 (n = 30), who recorded the lowest mean scores on economic misconceptions at the beginning of the semester and equal lowest at the end, did best on Part A of the examination, but fell behind in Parts B and C to students identified in Cluster 7 (n = 23) who, by comparison, held economic misconceptions that were higher, views on markets determining price that were also measured as high, and mean INT scores that were higher (and which increased across the semester). Despite this trajectory, Cluster 7 exhibits the highest marks on Parts B and C of the exam for reasons that can only be sought in additional sources of explanatory variation. At the other extreme of outcomes performance, the trajectory of Cluster 5 (n =11) is again unproblematic
Figure 3c, based on students with no prior knowledge of economics gained at school, reveals less variation on all of the economic prior-knowledge observables, although there are some changes across the semester on the INT cluster-mean scores (but generally less so than for students who had previously studied economics). From a teaching point of view there is, more encouragingly, some evidence to suggest that economic misconceptions decrease across the semester for some students in this subgroup. Again the extremes hold up well, as can be seen in the trajectory of the poor-performing Cluster 4 (n = 26) contrasted with the generally high-performing Cluster 3 (n = 30) for whom MKT increases, INT and EMC both decrease, and for whom MKT is generally much higher than both INT and EMC.
However, for the remainder, the inferred relationship here between economic misconceptions and final examination outcomes does appear to be generally less consistent. For example, and in contrast to the good performance of students in Cluster 3, students in the similarly profiled Cluster 1 perform somewhere ‘in the middle’.
In concluding the presentation of the cluster solutions, it should be noted that the modelling effects of two additional economic prior knowledge observables (EAC and ERE) have not been presented here, mainly because they add considerable additional graphical complexity to the analysis without providing substantively different insights.
Institutional responses in 2002
Partially as a response to these results, the integration of learning to learn exercises, instructor training, and feedback via the learning inventory, was further refined in 2002. As in 2001, students were asked to complete an inventory twice, the first time in the first 2 weeks of semester and again in the final 2 weeks. Unlike in 2001, however, in 2002 the first author (Shanahan) also gave a presentation to students in week 10, regarding the importance of the learning inventory and the gains that could be realised from discussing individual learning issues with a tutor as well as completing the inventory a second time.
The most important enhancement to the institutional responses made in 2002 was the development of a programme that created an immediate graphical representation of each individual student’s aggregated responses to the learning inventory. Colour-coded and labelled by category, these response sheets were produced and sent to the student the moment the individual submitted their responses to the learning inventory. Students were further asked to take their individual response sheet to their tutor in order to receive 1 per cent toward their final grade, and in the process (hopefully) to engage in a discussion with their tutor as to its contents and their meaning.
Further modifications were made to the learning exercises given to students. Rather than continue with individual exercises that served as the basis for regular tutorial discussion, groups of students were assigned the task of preparing and presenting work on their collective understanding of learning topics. In these group-based assignments (worth 5 per cent of a student’s total mark in the course), students were required to consider their responses to a collection of learning issues, again based on Biggs (1985), and present these to the rest of the class, producing both overheads and handouts. This approach (and the increased weighting in assessment) resulted in high-quality presentations and improved discussion compared to the individually based responses of the previous year.
The total weighting given to ‘learning to learn’ issues in the course therefore rose from a ‘bonus’ 4 per cent in 2001 to a maximum possible 7 per cent (5 per cent for the group presentation, and 1 per cent for each completed and submitted inventory) in 2002. This weighting, although still comparatively modest (for example, although the final examination was weighted at 60 per cent, students were still required to pass the examination to pass the course), appeared to increase student involvement significantly.
As in 2001, staff received instruction in interpreting and responding to variation in student learning from the second author (Meyer). In particular, advances in the design and quality of feedback to students, automatically generated on submission of the learning inventory, served as the focus for staff development. Increases in staff experience with the meta-learning approach (despite a turnover in tutors from the previous year of approximately 50 per cent) resulted in a higher awareness of variation in student learning among teaching staff and in the department as a whole.
Increased awareness by staff of variation in student learning (as opposed to the symptom – variation in student articulation of economic content) was reported, by tutors, to have increased the emphasis they placed on ‘how’ to learn as well as ‘what’ to learn, when teaching in tutorials. Whether this change produced any measurable influence on student learning (and outcomes) is the subject of continuing research.
Implications, future research and conclusions
Implications
The background to the results reported here includes an institution and course commitment to respond to variation in student learning. A prime objective is to assist students ‘at risk’ of failure, given their prior knowledge and preferred or habitual methods of learning engagement. The first step, partly reported here, is to identify variation in students’ prior knowledge of economic concepts. The second is to determine whether there is an association between these measures and student learning outcomes. The third step is to respond in a manner that, within the given constraints, allows staff to assist students in their learning and ultimately their learning outcomes.
A sustained programme of background research has isolated, and psychometrically refined, a set of measures that can be used to elicit variation in students’ conceptions of economic phenomena, three of which form the basis of the present study. Present findings suggest that these three relatively simple measures of prior knowledge are able to model, in a theoretically consistent manner, the tail ends of the learning outcome distribution(s) reflected in examination results. This is quite a remarkable and useful finding insofar as it can directly inform teaching responses, as well as student support, precisely because the variation in question is about economic phenomena rather than the perhaps less immediately accessible (to teachers and students) conceptual complexities of student learning engagement.
As a separate issue, the analytic approach taken produces a dynamic picture of prior knowledge that can be monitored across one (or more) semesters at a subgroup or even individual level. Such course feedback allows far deeper insights into students’ economic conceptions, and changes to these conceptions, than currently exists.
It is also clear that the capacity to model a wider range of learning outcomes using just the prior knowledge observables in the manner illustrated is less evident for students with no prior knowledge of the subject. Previous work (Meyer and Shanahan, 2001b) has confirmed that students entering the course with no such prior knowledge achieve statistically significant lower outcomes than students who studied economics at school. It may well be that learning outcomes need to be modelled quite separately for these two groups of students.
The partial results reported here do not suggest that existing institutional arrangements have made a measurable impact on students’ prior economic misconceptions across the full range of variability. This result might simply reflect the persistence of long-held views or beliefs about economic phenomena and an inability to alter them in a measurable way in just one semester. Alternatively, and less likely, it may be that current efforts at intervention are insufficient or misdirected.
Future research
There is a need to extend the present work to a wider diversity of students of economics. In addition to including students from other universities, there is a need to capture those students who, for whatever reason, have previously been excluded from the analysis – in particular, students who withdraw part-way through the course or who do not sit the final examination. Not only would such a wider sample of students allow further testing of the robustness of the insights provided here (especially the testing of sample bias); they would also provide avenues for further research, such as the association between inventory responses and withdrawal.
Another area of research is to examine more closely the relationship between current measures of student ability, such as (in South Australia) student entrance score and measures of learning engagement. While some attempts were made to address this research question several years ago (Cowie et al., 1997) there are now newer insights and more fully developed models of student learning engagement that can be employed.
The measuring instrument itself is also subject to ongoing refinement. For example, it is possible that one reason why students’ conceptions of price become more equivocal over time is that they actually gain a deeper understanding of the complexity of economics during the semester, and become less certain about ‘simple’ single-statement responses as a consequence. A similar result may hold for students who have had prior exposure to economics (at school perhaps) before entrance to university. There is need, therefore, to further refine statements and the conclusions that can be drawn from students’ responses to these.
A closer examination of ‘levels’ of English ability would provide a finer-grained insight into what is currently a relatively blunt indicator of student language status. There is a need to align outcome measures more closely with their conceptual learning analogues (for example, the capacity of multiple-choice questions to capture variation in deep-level learning outcomes). Increased partitioning of students (by age, previous learning experiences of all kinds, subject majors, and so on) would also be useful. There is also a need to conduct longitudinal studies over a longer interval, especially across an entire degree programme.
Conclusions
The relatively simple models that have been considered have revealed some powerful and tantalising insights – in particular, the potential for a single measure such as that used here to capture variation in ‘economic misconceptions’ to identify students at the upper and lower extremes of the distribution of final examination results. Nonetheless, the exclusive use of such a simple measure is not advocated. Underlying the apparently simple ‘boundary measures’ is a complex architecture of learning engagement, levels of student prior knowledge and other factors not presented here, which all impact on learning outcomes.
The results also provide a reflective opportunity for staff engaged in teaching economics to first-year students. Not only do some students arrive with measurably lower levels of misconceptions than others; some of these students actually perform poorly in examination after a semester’s exposure to the subject. While there may be several reasons for this observation (from jaded student responses to learning inventories, to poor teaching), it is distressing that many students with prior knowledge of economics do worse at the end of a semester than those with no such prior knowledge. It is also worth observing that there is no obvious reason why first-year economics is unique among university courses in having students who arrive with misconceptions, or having some of these students perform worse on a ‘misconceptions’ measure at the end of a semester. To our knowledge, however, it is comparatively unusual for a first-year course to be attempting to detect and respond to these issues.
More broadly, our findings may also be suggestive of the need to reconsider current trends in higher education. For example, current trends towards modularisation of programmes and efforts to broaden students’ education, while important for flexibility in the delivery of education and the overall development of the student, may be occurring at the cost of producing a deeper transformation in students’ conceptions. For example, in many discipline areas the ultimate aim is to produce students who ‘think like an economist’, or ‘think like a historian’, or ‘see through a scientific lens’, and so on, to use popular metaphors. Such aims may in fact be made less attainable where programmes are increasingly fragmented and diversified. For some students there is a persistence of prior knowledge and, to the extent that it can be measured, it appears here to be resilient to change over a single semester. Whether, at the end of a 3-year programme, students’ views have been influenced, or whether current practices merely select those students whose prior conceptions are compatible with those in the discipline, remains the subject of ongoing research.
Appendix 1 Explanation of economic subscales
Adapted from Meyer and Shanahan (1999, 2002).
- An economic misconceptions subscale (EMC), defined by a group of eight items (average alpha = 0.66) that identified student perceptions regarding the aims, objectives and limitations of the discipline of economics. In this category, students were asked to respond to statements such as ‘economics is an exact science’ and ‘economics is the study of money’.
- A subscale representing economist activity (EAC) (essentially what an economist does), defined by statements that focus on the role of economists and economics in society (average alpha = 0.56). For example, students were asked to respond to the statement that ‘an economist determines what acceptable levels the economy should be at’ and ‘an economist studies people and how they make choices’.
- An economic reasoning subscale (ERE), defined by statements relating to the underlying approach (or paradigm) of economics (average alpha = 0.53). Responses are intended to reveal whether a student has an interest in, and an affinity for, economic reasoning. An example of a statement in this category is ‘I often wonder what the economic reasons are behind newsworthy events’ and ‘in overlooking an instance of unacceptable social behaviour (for example, verbal abuse) I often ask myself what the world would be like if everybody behaved like that’.
- A fourth structure also emerged representing variation in the concept of economic worth (price determination), based on an attempted operationalisation of qualitative variation in students’ conceptions of ‘worth’ and ‘price’ after the phenomenographic study of Dahlgren (1984). Students responded to statements such as ‘the cost of manufacturing a product (for example, a loaf of bread) determines its worth’ and ‘what you pay for something is what it is worth’. Agreement with the former implies that ‘worth’ (price) is determined by aggregating the costs of production, while agreement with the latter statement implies that ‘worth’ (price) is determined by supply and demand. Two subscales, one assessing dimensions of students’ conceptions of the market influence on price (MKT) and the other views on the intrinsic worth of a good and its price (INT), were formed.
Contact details
Martin P. Shanahan
University of South Australia
School of International Business
North Tce, Adelaide, 5000
South Australia, Australia
Email: martin.shanahan@unisa.edu.au
Jan H. F. Meyer
University of Durham
University of South Australia
University of Durham, School of Education
Leazes Road, Durham DH1 1TA
United Kingdom
Email: j.h.f.meyer@durham.ac.uk
References
Biggs, J. B. (1985) ‘The role of meta-learning in study processes’, British Journal of Educational Psychology, vol. 55, pp. 185–212.
Cowie, J., Shanahan, M. and Meyer, E. (1997) ‘Measuring learning processes in first year economics: preliminary results’, Research and Development in Higher Education, vol. 20, pp. 209–30.
Dahlgren, L. O. (1984) ‘Outcomes of learning’, in F. Marton , D. Hounsell and N. Entwistle (eds), The Experience of Learning, Edinburgh: Scottish Academic Press.
Meyer, J. H. F. (1999) ‘Assessing outcomes in terms of the “hidden” observables’, in C. Rust (ed.), Improving Student Learning – Improving Student Learning Outcomes, Oxford: OCSD/Oxford Brookes University.
Meyer, J. H. F. (2000a) ‘An empirical approach to the modelling of “dissonant” study orchestration in higher education’, European Journal of Psychology of Education, special issue, vol. 15, pp. 5–18.
Meyer, J. H. F. (2000b) ‘Embryonic “memorising” models of student learning’, Educational Research Journal, vol. 15, pp. 203–21.
Meyer, J. H. F. (2000c) ‘Variation in contrasting forms of “memorising” and associated observables’, British Journal of Educational Psychology, vol. 70, pp. 163–76.
Meyer, J. H. F. and Boulton-Lewis, G. M. (1999) ‘On the operationalisation of conceptions of learning in higher education and their association with students’ knowledge and experiences of their learning’, Higher Education Research and Development, vol. 18, pp. 289–302.
Meyer, J. H. F. and Shanahan, M. P. (1999) ‘Modelling learning outcomes in first-year economics’, paper to 8th European Conference for Research on Learning and Instruction, Göteborg, Sweden, 24–8 August.
Meyer, J. H. F. and Shanahan, M. P. (2001a) ‘Making teaching responsive to variation in student learning’, in C. Rust (ed.), Improving Student Learning 8: Improving Student Learning Strategically, Oxford: OCSD/Oxford Brookes University.
Meyer, J. H. F. and Shanahan, M. P. (2001b) ‘A triangulated approach to the modelling of learning outcomes in first year economics’, Higher Education Research and Development, vol. 20, no. 2, pp. 127–45.
Meyer, J. H. F. and Shanahan, M. P. (2002) ‘On variation in conceptions of “price” in economics’, Higher Education, vol. 43, pp. 203–25.
Meyer, J. H. F. and Vermunt, J. (guest eds) (2000) ‘Dissonant study orchestration in higher education: manifestation and effects’, European Journal of Psychology of Education, special issue 15.
Shanahan, M. P. and Meyer J. H. F. (2001) ‘A student learning inventory for economics based on the students’ experience of learning: a preliminary study’, Journal of Economic Education, vol. 32, no. 3, pp. 259–67.
Notes
[1] This research was supported by a University of South Australia Teaching and Learning Grant. The authors would like to acknowledge the assistance of the University of South Australia Dean of Teaching and Learning, Vicki Feast; Pro Vice-Chancellor, Kevin O’Brien; their teaching colleagues Ken Adams and Gerald McBride; and tutors Tarnia Roberts, Arthur Kiriakis, Mark Jackson, Ian North and Marni Mead. Participants at the 9th European Conference for Research on Learning and Instruction, Fribourg, Switzerland, 28 August–1 September 2001 and two referees provided helpful comments on an earlier version of this paper.
[2] The term learning history refers to a student’s most recent previous approaches to, and experiences of, learning engagement as contextualised, for example, at school or in the workplace. To the degree that this history (especially habitual or preferential aspects of it) is likely to be transferred to new contexts together with beliefs about what ‘learning’ is, it is crucial in understanding a student’s likely predisposition to engage subsequent learning episodes.
[3] The impact of prior mathematical attainment on student success is also a function of the quantity and quality of the mathematical content expected in the course. In the work reported here, including the work across two universities (Meyer and Shanahan 1999), all students completed introductory first-year economics courses of a similar nature that did not differentiate between students majoring in economics and those majoring in more general business or commercial studies. Apart from basic algebra and graphing skills, little mathematical content was evident in any of the courses taught at this level.